Leveraging Cloud-Based Data Science Solutions: Pros and Cons
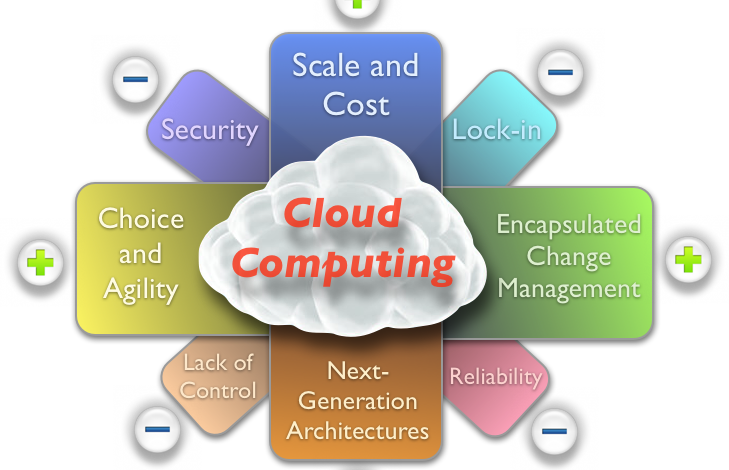
Introduction
Cloud-based data science solutions have revolutionised how organisations handle, process, and analyse large datasets. By utilising cloud computing, businesses can scale their operations, access powerful computational resources, and collaborate more efficiently. However, like any technology, it comes with its advantages and challenges. Exploring the key pros and cons of cloud-based data science solutions is quite a useful career-enhancing exercise, especially for urban professionals looking to enhance their technical base by enrolling in a data science course in Kolkata and such cities.
Pros of Cloud-Based Data Science Solutions
Organisations adopt cloud-based data science solutions mainly for scalability, cost efficiency, and real-time collaboration. These platforms offer flexible computing power, secure data storage, and seamless integration with advanced analytics tools, driving faster, more informed decision-making and innovation. These advantages of adopting cloud computing and shifting to cloud platforms are motivating an increasing number of businesses to adopt cloud-based data science solutions..
Scalability and Flexibility
Cloud platforms offer elastic computing resources that can scale with demand. This means you can start small and ramp up as your data science needs grow, without having to invest in additional hardware. For those pursuing a data science course, understanding cloud scalability can provide a major competitive edge in building solutions for dynamic workloads.
Example: AWS, Google Cloud, and Azure allow auto-scaling of resources, making it easy to manage fluctuating workloads.
High Computational Power
Cloud services offer access to cutting-edge hardware such as GPUs and TPUs, which are essential for complex machine learning and deep learning tasks. These resources are often prohibitively expensive for smaller organisations to purchase outright.
Example: Cloud providers offer specialised environments optimised for AI and machine learning workloads, speeding up the development of models.
Cost-Efficiency
With cloud-based solutions, you pay only for the resources you use. This eliminates the need for upfront capital expenditures on infrastructure. Additionally, cloud vendors often offer pay-as-you-go pricing models, helping organisations better manage their budgets. Learning to implement these cost-effective strategies in a data science course can be invaluable for both individuals and businesses.
Example: Instead of purchasing expensive servers, you can rent cloud storage and computing power at a fraction of the cost.
Automated Maintenance and Security
Cloud providers typically manage infrastructure maintenance, updates, and security patches, ensuring systems remain up-to-date. This reduces the burden on in-house IT teams and helps maintain compliance with data protection standards.
Example: Major cloud vendors invest heavily in security, providing robust solutions for data encryption and backup.
Collaboration and Accessibility
Cloud-based platforms enable teams to collaborate seamlessly by sharing data, models, and code from anywhere in the world..
Example: Platforms like Databricks and Google Colab allow multiple users to work simultaneously on data science notebooks, enhancing teamwork.
Cons of Cloud-Based Data Science Solutions
The challenges associated with adopting cloud-based data science solutions are quite a few. While high costs and security concerns are the primary issues, there are a few others too. Here is a summary.
Data Privacy and Security Concerns
While cloud vendors provide security features, some organisations may have reservations about storing sensitive data in the cloud due to potential breaches or unauthorised access. Regulatory concerns may also limit the type of data that can be stored on public cloud platforms.
Example: Industries like healthcare and finance must comply with strict data regulations (e.g., HIPAA, GDPR), which could limit their cloud usage.
Dependence on Internet Connectivity
Cloud-based solutions require stable and fast internet connections. For organisations with unreliable internet, this can disrupt operations, especially during critical phases of data analysis or model training.
Example: If internet connectivity fails during a large-scale data processing task, it could lead to delays or data loss.
Ongoing Costs
While cloud solutions offer cost-saving benefits initially, they can become expensive over time as data storage and computational needs grow. If not managed carefully, cloud bills can quickly escalate, making it crucial to optimise resource usage. Reducing cost overheads is a tricky area for those working on cloud-based data science projects. This issue often requires the intervention of experts, and is a topic covered in any data science course that covers cloud-based solutions.
Example: Continuous data storage or running large-scale machine learning models can lead to unexpected costs if usage isn’t monitored regularly.
Limited Customisation
Cloud-based solutions often come with predefined configurations and services. For organisations with specific needs, this may limit their ability to customise their data science environments or tools.
Example: Some businesses might require specialised configurations that cloud platforms may not natively support, limiting their operational flexibility.
Data Transfer and Latency Issues
Transferring large datasets to the cloud can be time-consuming, especially for organisations dealing with terabytes of data. Latency issues may arise if the data needs to be accessed frequently or if there are limitations in network bandwidth.
Example: Uploading and retrieving vast datasets from the cloud might cause delays in real-time analytics.
Conclusion
Cloud-based data science solutions offer undeniable advantages, especially for scalability, collaboration, and cost-efficiency. However, organisations must carefully weigh the pros and cons to ensure they meet their data security needs, budget constraints, and customisation requirements. By understanding these trade-offs, businesses, and professionals can make studied decisions regarding migrating their business processes to the cloud. Urban professionals can enrol in a specialized technical course such as a data science course in Kolkata that will equip them to evaluate the pros and cons of migrating to the cloud and make informed decisions about how to best leverage the cloud for their data science initiatives.
BUSINESS DETAILS:
NAME: ExcelR- Data Science, Data Analyst, Business Analyst Course Training in Kolkata
ADDRESS: B, Ghosh Building, 19/1, Camac St, opposite Fort Knox, 2nd Floor, Elgin, Kolkata, West Bengal 700017
PHONE NO: 08591364838
EMAIL- [email protected]
WORKING HOURS: MON-SAT [10AM-7PM]
DIRECTIONS:
https://maps.app.goo.gl/opJzZwixnf3hcdLf9